Building explainability into the components of machine-learning models
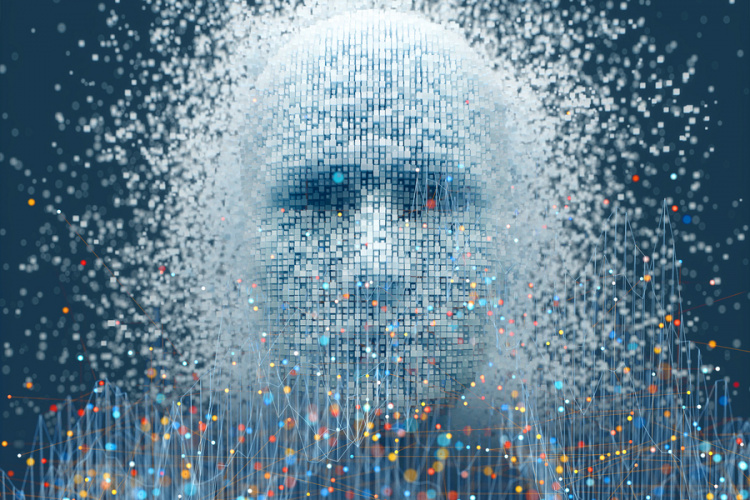
Explanation methods that help users understand and trust machine-learning models often describe how much certain features used in the model contribute to its prediction. For example, if a model predicts a patient’s risk of developing cardiac disease, a physician might want to know how strongly the patient’s heart rate data influences that prediction.
But if those features are so complex or convoluted that the user can’t understand them, does the explanation method do any good?
MIT researchers are striving to improve the interpretability of features so decision makers will be more comfortable using the outputs of machine-learning models. Drawing on years of field work, they developed a taxonomy to help developers craft features that will be easier for their target audience to understand.
“We found that out in the real world, even though we were using state-of-the-art ways of explaining machine-learning models, there is still a lot of confusion stemming from the features, not from the model itself,” says Alexandra Zytek, an electrical engineering and computer science PhD student and lead author of a paper introducing the taxonomy.
https://news.mit.edu/2022/explainability-machine-learning-0630
Your Comment :